Home < Technology
Intelligent load management for a sustainable, innovative and future-proof grid
Our software creates a digital twin of the thermal network and optimizes operations through improved load management: peak loads are smoothed out, temperatures are reduced and costs are lowered as a result. Different energy sources can be linked and dynamic energy prices can be integrated.
It automatically learns the thermal properties and energy requirements across the network and ensures efficient operation.
Home < Technology
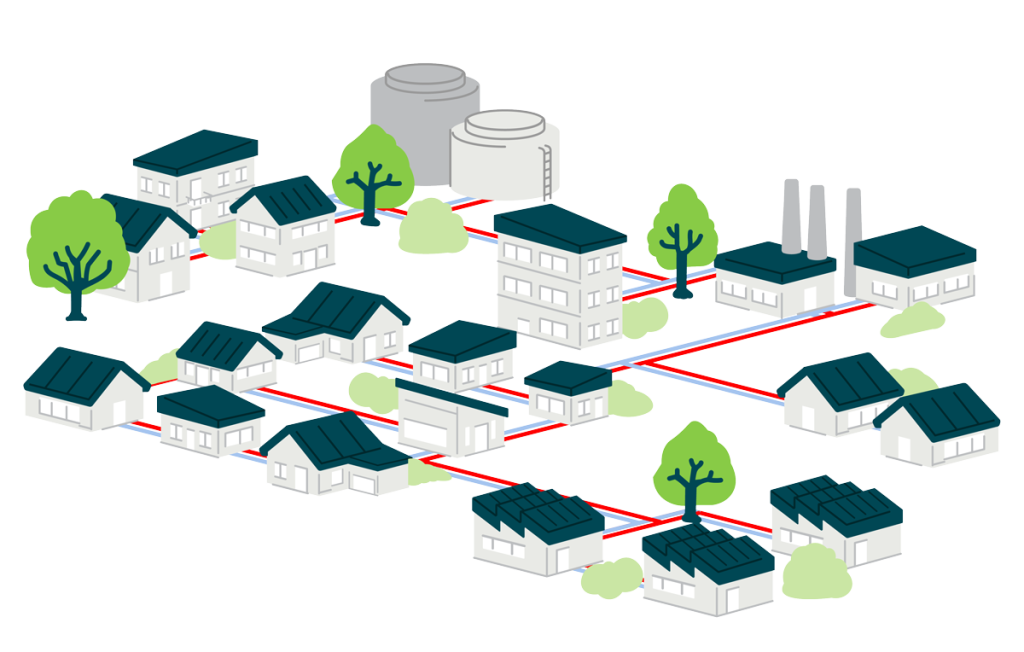
Intelligent load management
Load management in intelligent heating networks actively controls energy demand and distribution by partially shifting consumption. Knowledge of the network characteristics and the exact heat demand of the consumers is crucial for optimization.
Our software creates digital twins in real time and enables active monitoring and control of the thermal properties of the distribution grids and the connected buildings.
This allows load peaks to be smoothed out, heat sources to be optimally utilized, inactive buildings to be used as energy storage and local water reservoirs in the network to be actively managed.
Before
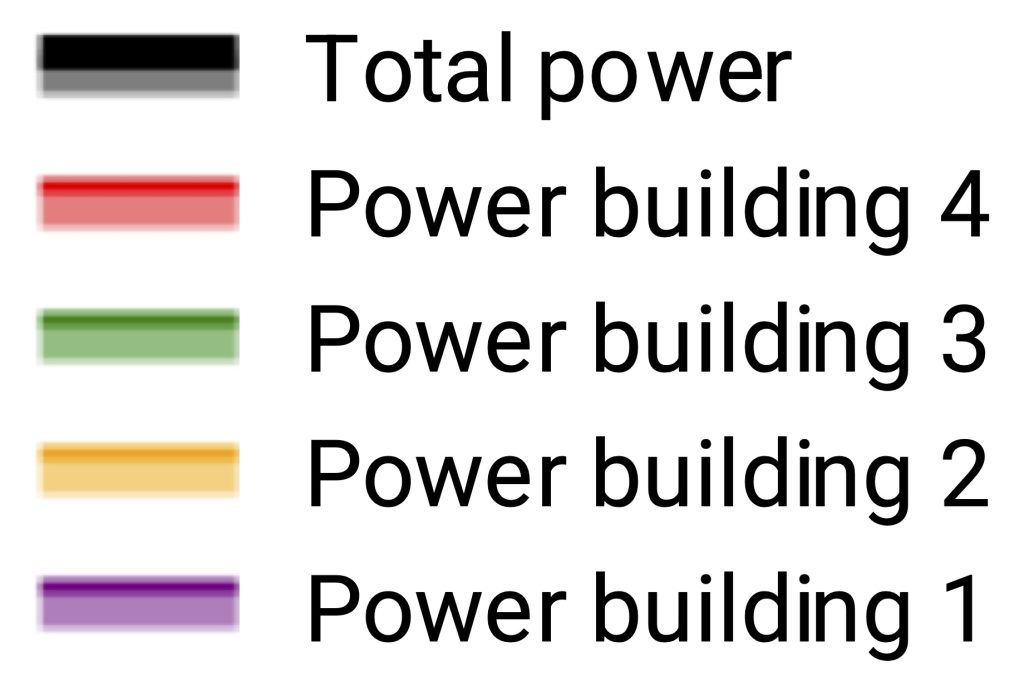
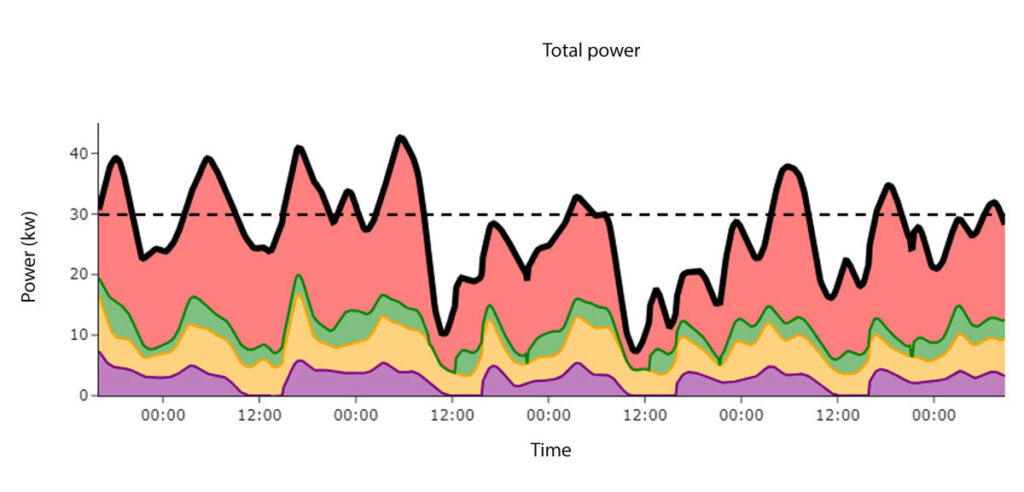
After
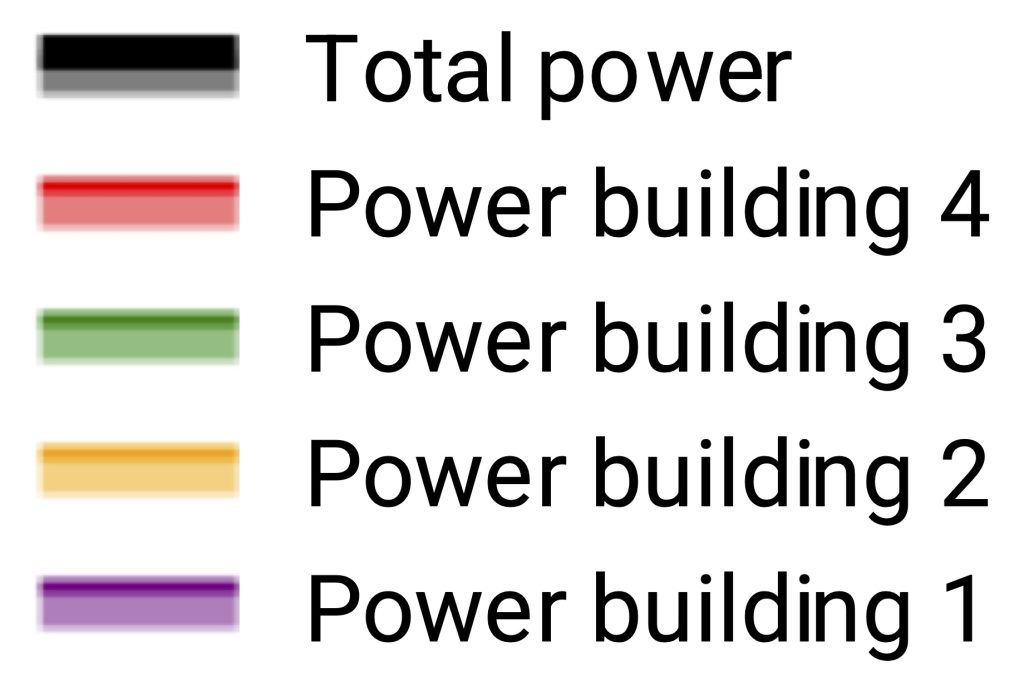
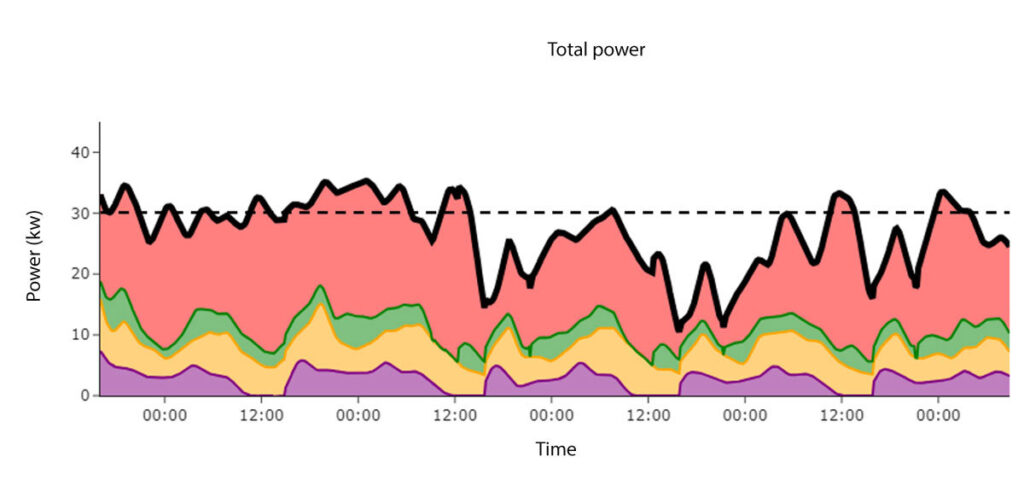
These key factors drive optimization success
Intelligent load management is made possible by taking a holistic view of the grid and is made up of a combination of individual measures or connection points, whereby the implementation of even one measure enables more efficient and ecological management.
1. Optimization of Boiler Runtime
Through load forecasting, the management of the storage in the central unit can be optimized by the heating boiler so that, for example, the wood boiler runs for as long as possible. This minimizes the number of on/off cycles during transition periods and summer.
2. Optimization of flow and return temperatures
Adjusting the flow temperatures based on the current and forecast demand also optimizes the return temperatures and can lead to considerable reductions in thermal losses in the network and energy savings.
3. Grid Optimization / Utilization of Network Capacity
The piping in district heating networks contains a significant volume of water, which has a non-negligible thermal capacity. Our optimization procedures deduce the optimal preheating strategy of pipes to smoothen peaks loads.
4. Optimal Use of Decentralized Thermal Storage
Decentralized thermal storage units and hot water boilers are used to distribute and store excess heat in the network and between buildings. When required, the heat is only released in places where it is actually needed.
5. Active Use of the Thermal Capacity of the Buildings
The heat capacity of the connected buildings is significantly larger than that of buffer storage. Our algorithms actively use this capacity to smooth short-term peak loads.
Modern technology enables the realisation
Model Predictive Control
(MPC)
We predict the heat requirements in real time and control the grid to satisfy user heat requirements while minimizing losses. Our prediction algorithms make use of historical on-site data, on-site weather forecasts and internal know-how of other similar grids.
Digital twin
We create a digital twin of each building and the entire network, including real-time thermodynamics of each connected component. Precise real-time monitoring allows us to quickly respond to changes in requirements. It also allows us to automatically detect long-term changes in thermal properties of components, report potential leaks and sub-optimal components.
Artificial intelligence
(AI)
Our forecasting and control algorithms make use of state-of-the art machine learning and AI technologies to detect complex patterns in user day-to-day heat requirements, improving efficiency of our predictive heating strategies and saving energy.